What is clustering in AI?
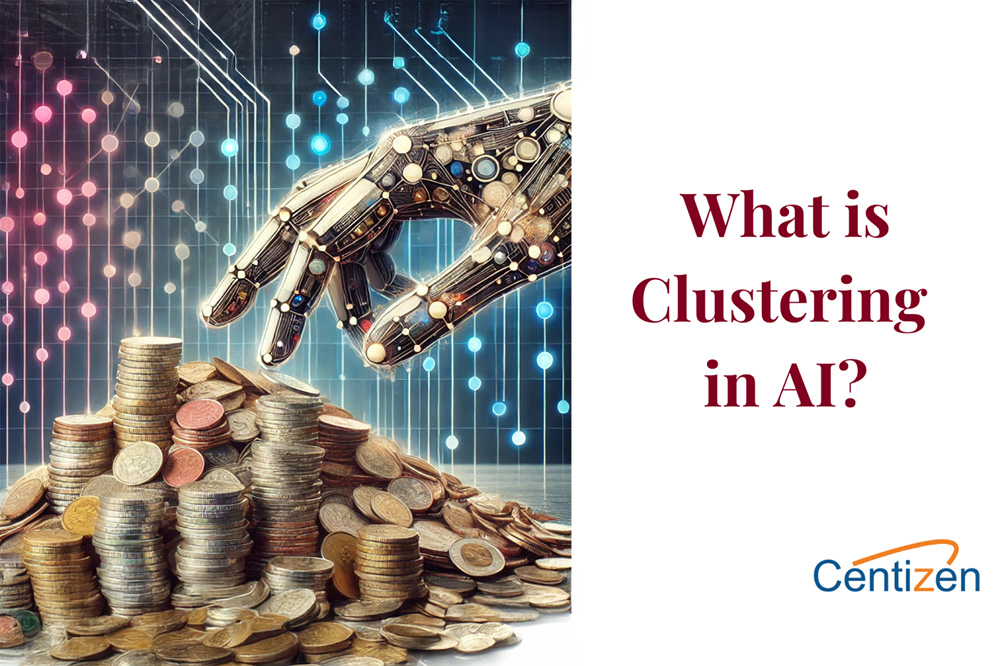
Clustering is a fundamental concept in Artificial Intelligence (AI) and Machine Learning that enables AI to group similar data points without prior labels. It’s like sorting a pile of mixed coins into different categories based on their size and color—without being told which ones are pennies, nickels, or dimes.
In this article, we’ll break down what clustering is, how it works, and its real-world applications in AI.
1. What is clustering?
Clustering is a type of unsupervised learning, meaning AI identifies patterns without predefined labels. It finds hidden structures in data by grouping similar items together.
Example: Imagine an AI analyzing customer shopping habits. Instead of manually labeling customers, the AI groups them based on behavior—frequent buyers, occasional shoppers, and one-time visitors.
This helps businesses personalize marketing, predict trends, and optimize customer service without predefined categories.
2. How does clustering work?
1. Data input
- The AI receives raw data with no predefined labels.
- Example: Customer purchase history, images, or user activity logs.
2. Grouping similar data
- The AI analyzes data points and identifies similarities.
- Example: Customers with similar buying patterns get grouped together.
3. Cluster formation
- AI divides data into clusters where similar points are placed together.
- Example: An AI groups movies based on audience preferences.
4. Insights & decision-making
- The identified clusters help businesses, researchers, or systems make better decisions.
- Example: Retailers offer discounts to frequent shoppers based on their cluster.
3. Why is clustering important?
Clustering helps AI:
- Find hidden patterns: AI groups data without human-defined categories.
- Improve personalization: Businesses use clustering to offer better recommendations.
- Detect anomalies: Unusual patterns can indicate fraud or security threats.
- Organize information: AI sorts search results, news articles, or documents efficiently.
4. Real-world applications of clustering
- Marketing & customer segmentation: AI groups customers by behavior for targeted ads.
- Healthcare: AI clusters patient symptoms to detect disease patterns.
- Fraud detection: Banks use clustering to flag suspicious transactions.
- Search engines: AI organizes and ranks search results based on similarity.
- Image recognition: AI clusters visually similar images in large datasets.
5. Challenges of clustering
While clustering is powerful, it has limitations:
- Choosing the right number of clusters: Too many or too few can reduce accuracy.
- Handling complex data: AI may struggle with overlapping clusters.
- Data quality dependence: Poor-quality data affects clustering accuracy.
Conclusion
Clustering is a powerful AI technique that helps systems find structure in unstructured data. From customer segmentation to fraud detection, clustering enables AI to make sense of large datasets without human guidance.
Our services:
- Staffing: Contract, contract-to-hire, direct hire, remote global hiring, SOW projects, and managed services.
- Remote hiring: Hire full-time IT professionals from our India-based talent network.
- Custom software development: Web/Mobile Development, UI/UX Design, QA & Automation, API Integration, DevOps, and Product Development.
Our product:
- ZenBasket: A customizable ecommerce platform.
- Zenyo payroll: Automated payroll processing for India.
- Zenyo workforce: Streamlined HR and productivity tools.
Centizen
A Leading IT Staffing, Custom Software and SaaS Product Development company founded in 2003. We offer a wide range of scalable, innovative IT Staffing and Software Development Solutions.
Contact Us
USA: +1 (971) 420-1700
Canada: +1 (971) 420-1700
India: +91 63807-80156
Email: contact@centizen.com
Our Services
Products
Contact Us
USA: +1 (971) 420-1700
Canada: +1 (971) 420-1700
India: +91 63807-80156
Email: contact@centizen.com