What is Dimensionality Reduction in AI?
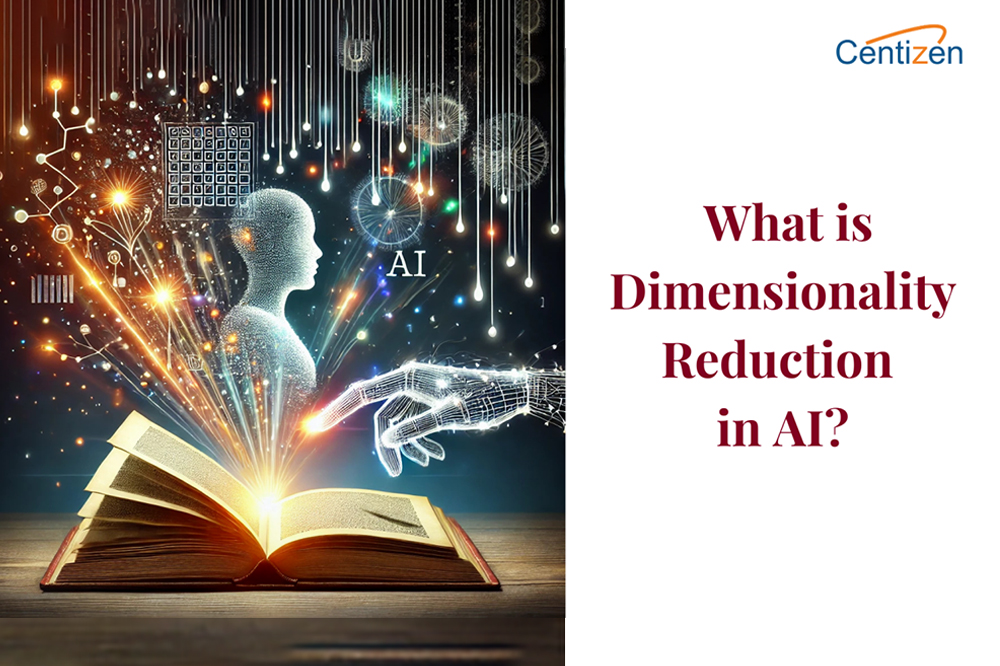
AI models process massive amounts of data, but sometimes too much data can be a problem. Imagine trying to understand a 1,000-page book—if only 100 pages contain the important details, reading the entire book would be inefficient.
That’s where Dimensionality Reduction helps! It removes unnecessary data while keeping only the most useful information, making AI smarter, faster, and more efficient.
In this article, we’ll break down what dimensionality reduction is, why it matters, and how it’s used in AI.
1. What is dimensionality reduction?
Dimensionality Reduction is a technique in AI and Machine Learning that removes irrelevant or redundant features from a dataset while keeping the essential information.
Instead of working with thousands of features, AI models can focus on a smaller set of important features, improving performance.
Example: A facial recognition AI might analyze 1,000 facial features, but only a few dozen (like eye shape, nose width, and jawline) are critical for accurate identification. Dimensionality Reduction helps eliminate unimportant features, making recognition faster and more efficient.
2. Why is dimensionality reduction important?
- Speeds up AI training – AI models process data faster when there are fewer unnecessary features.
- Improves accuracy – Too much data can confuse AI; reducing it helps focus on what matters.
- Prevents overfitting – If AI learns too many details, it may memorize rather than generalize.
- Reduces computational cost – Less data means less processing power required.
3. How does dimensionality reduction work?
There are two main techniques used in Dimensionality Reduction:
1. Feature selection
- AI picks only the most relevant features and removes unnecessary ones.
- Example: In medical diagnosis, an AI may remove irrelevant data (e.g., patient’s name) and focus on blood test results.
2. Feature extraction
- AI combines multiple related features into a smaller set of meaningful features.
- Example: In image processing, thousands of pixels can be grouped into larger patterns instead of analyzing each pixel separately.
4. Common dimensionality reduction methods
- Principal component analysis (PCA) – Finds patterns in data and reduces dimensions while keeping important information.
- t-SNE (t-distributed stochastic neighbor embedding) – Helps visualize high-dimensional data in 2D or 3D.
- Autoencoders – Uses deep learning to encode and compress data efficiently.
5. Real-world applications of dimensionality reduction
- Healthcare – Reduces unnecessary patient data, keeping only vital symptoms for disease diagnosis.
- Facial recognition – Focuses on key facial features instead of processing every tiny detail.
- Stock market prediction – Filters out noise and keeps only essential economic indicators.
- Customer segmentation – Groups customer preferences efficiently for targeted marketing.
Conclusion
Dimensionality Reduction is like summarizing a book—you remove unnecessary details while keeping the key points. By reducing data complexity, AI models become faster, more efficient, and better at decision-making.
Our services:
- Staffing: Contract, contract-to-hire, direct hire, remote global hiring, SOW projects, and managed services.
- Remote hiring: Hire full-time IT professionals from our India-based talent network.
- Custom software development: Web/Mobile Development, UI/UX Design, QA & Automation, API Integration, DevOps, and Product Development.
Our product:
- ZenBasket: A customizable ecommerce platform.
- Zenyo payroll: Automated payroll processing for India.
- Zenyo workforce: Streamlined HR and productivity tools.
Our Services
Products
Contact Us
USA: +1 (971) 420-1700
Canada: +1 (971) 420-1700
India: +91 63807-80156
Email: contact@centizen.com
Centizen
A Leading IT Staffing, Custom Software and SaaS Product Development company founded in 2003. We offer a wide range of scalable, innovative IT Staffing and Software Development Solutions.
Contact Us
USA: +1 (971) 420-1700
Canada: +1 (971) 420-1700
India: +91 63807-80156
Email: contact@centizen.com